Experimenting With Daily Email - Which One Performs Better?
Mortgage rates move daily, and we like to keep our customers aware of them, but does awareness drive conversion?Our mission at Better is to provide Borrowers with the best mortgage and the best borrowing experience possible. One unique aspect of our work is that mortgage rates move daily, and we do our best to keep our Borrowers appraised of market conditions on a variety of cadences using a few different emails. As opposed to "re-engagement" emails a borrower might receive from other companies, our emails actually contain time-sensitive information that might save them tens of thousands of dollars in the long run.
One of these emails is the Daily Rate Tracking Email, which predates most of our employees and shows Borrowers a simple table of rates they qualify for. The team's going assumption has been that this email should drive some noticeable level of conversion, since it contains actionable information about significant potential savings.
Seeing as we have experienced explosive growth over the last year, with a quadrupling in size of our product, design, data, and engineering organization (we're hiring!), our Borrower Experience squad decided to re-visit this assumption.
Before we get into the results of the Rate Tracking Email test, let’s talk a bit about Kansas.
Kansas /ˈkænzəs/ is a non-mountainous U.S. state in the Midwestern United States.
This is a picture of a highway. In Kansas.
What does a highway in Kansas have to do with Rate Tracking Emails?
Good question.
There are a couple similarities between this highway in Kansas, and the results of our multivariate Rate Tracking Email test.
What are those similarities ?
For one, Kansas is pretty flat. What’s also flat? The conversion impact of these Rate Tracking Emails.
How so?
The control and 2 variants of this experiment (showAll, showNone) turned out to have a very similar app to lock conversion rate.
The chart above is a "Kaplan-Meier curve" which charts conversion rate as a function of hours since exposure. What it's basically saying is that it is unlikely that any of the variants helped our application to "lock" conversion goal in any significant way.
If you look closer at the lines in the graph, one may conclude that the ‘control’ group performs higher - but that interpretation wouldn’t necessarily be correct. The fact that the shaded area of the lines are overlapping means that the 3 variants are not all that different.
Those shaded areas are known as the confidence intervals. More on that here.
Cool. What else about Kansas?
The second similarity is that the Kansas highway picture seems to lead to nowhere. What’s also going nowhere?
Potential Borrowers. After they receive these Rate Tracking Emails.
Huh?
Well, at least the emails in their current form.
One of the variants we tested included turning off the feature completely, aka showNone.
The way this variant would work is that we would turn off the ability for Borrowers to sign up or receive rate tracking emails completely. In other words, they would never be exposed to this feature, nor be aware that it was something they were missing.
The original hypothesis behind the showNone variant was:
We believe that Borrowers at this stage in the funnel are overwhelmed by the amount of emails and phone calls that they receive from Better, and this rate tracking email, specifically, does not encourage action.
Our prediction was:
By not sending a rate tracking email, Borrowers will be more inclined to login and check their rates, which will ultimately lead these Borrowers to be more engaged, and convert to lock at a higher rate compared to the variant that receives the daily emails (control, showAll)
But that prediction was false too.
So… what’s the point of all this?
The point is, when we asked our internal team, the experts, the following question:
Which rate tracking option do you think will have the highest impact on a Borrower’s app to lock conversion?
Predictions | Votes |
---|---|
A. control - Rate tracking email (shows 3-4 options around PAR rate) | 0 votes |
B. showAll - Rate tracking email with all rates | 9 votes |
C. showNone - No email. remove functionality. | 1 vote |
D. No noticeable change between options A through C | 0 votes |
And the answer was:
Results |
---|
D. No noticeable change between options A through C |
The answer was D. No noticeable change. Flatter than Kansas. Everyone was wrong.
Whoa, 10 out of our 10 experts predicted this outcome incorrectly?
Yes. But this isn’t surprising. There are a couple hypotheses that could help explain this:
- Hypothesis #1 - Because no one complained about it, we assumed the original feature was good (control variant)
- When our team started here, the rate tracking feature already existed. The person that wrote the original code left the company, and it was a non-trivial task to determine how the feature worked. Perhaps because the code was complicated, we assumed it was still performing as well as it did when it was built.
- These emails are system-generated by a tool our engineering team uses called Mandrill. Our Marketing org does not use Mandrill, but instead uses a tool called customer.io. I am certain that if our wonderful colleagues in Marketing had visibility into these emails via customer.io or another more user-friendly tool, these emails would have been fixed.
- Hypothesis #2 - Some comms could be detracting from the user experience
- Borrowers seem to want to know a lot about rates - but is a daily email the right method to communicate this? Do Borrowers actually want emails? Do they want any of the communications we are sending them? Maybe we should text them? Maybe there should be an app they could check everyday? All valid questions that should be asked.
- Hypothesis #3 - The phrasing and implication of my survey to the experts caused a bias in responses
- No doubt. As with all sorts of questioning and tests, there exists bias. We do our best to try and eliminate any sort of bias when experimenting, but as you can see from this list - there are a lot of them
So, what should we do with these emails?
From an app to lock perspective, it doesn’t matter. Leaving them on or off doesn’t move the needle.
But, being more proactive, there are a ton of things we can do with this new learning:
- We can do more heavy analysis.
- Try and understand if we tested correctly, or had bad data, or inadvertently introduced some sort of bias in the experiment.
- We could continue to experiment with optimizations.
- We could change the subject line of the email to see if more people will open it. We just tested the quantity of rates - not the copy, content or messaging of the emails.
- We could let the experiment run even longer.
- Maybe more experiment entrants will reveal a winner.
- We could turn them off.
- Given these emails are not providing any noticeable value, we probably could earn some Borrower goodwill back by turning these daily emails off.
- We could turn these emails over to the Marketing org to work their magic and decide their fate
- For some reason that predates most of the team, these emails were built in Mandrill. Mandrill doesn’t integrate nicely with the other tools we use for Marketing emails.
- Our marketing team are experts at this, and they should be empowered to make these decisions.
Key Learnings:
Often times, as a PM in a new company, I end up inheriting someone else’s compliance requirement, wonky process, or a great must-have feature.
Those inherited requirements may have been must-have and brilliant at some point, but these sort of things eventually go stale. Requirements should be revisited and validated every planning cycle to ensure they still make sense.
Next time I inherit a task, feature, or someone else’s idea - I’ll trust that it may have been great at one point, but will verify to see if it still makes sense.
Said more simply — trust, but verify.
Special thanks to everyone who worked on this: Marshal Bratten, Aidan Woods, Kiran Dhillon, Abhijith Reddy, Koty Wong, Paul Veevers, Meg McGrath Vaccaro, Felicity Wu, George Chearswat, Sabrina Scandar, Nam Gyeong Kim, and Josh Stein.
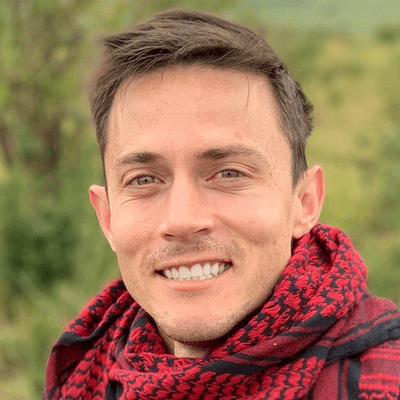
Our thinking
Wizard — our ML tool for interpretable, causal conversion predictions
Building an end-to-end ML system to automatically flag drivers of conversionFri Dec 27 2019—by Kenny Ning6 min read- data
- python
Engineering a Diverse Team: Interview with Sonya Chhabra, Part II
Today we’re continuing our conversation with Sonya Chhabra, an Engineering Manager who joined Better in the summer of 2020. This part of the discussion focuses on diversity in tech and Sonya’s experiences as a woman in tech.Tue Sep 28 2021—by Sonya Chhabra8 min readLooking for computer vision summer interns
Mon May 21 2018—by Erik Bernhardsson2 min read- internship
- machine learning